Is Your Hotel Ready for Business Intelligence?
Read More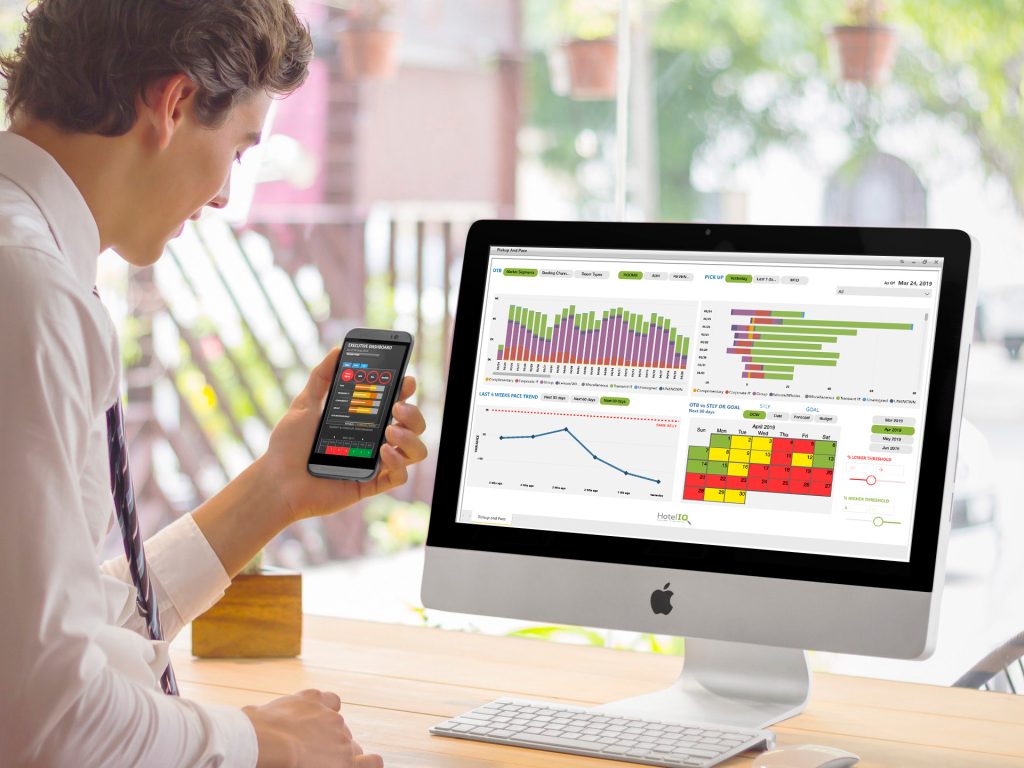
Business Intelligence (BI) is the process that is at the core of the “big data” articles and discussions that you have likely read about in the hospitality press this year. And while “big data”, as the name implies, refers to harnessing information from massive amounts of data at the enterprise and industry levels, the core business intelligence processes and technologies supporting big data can and should be focused on individual business units – in our case, hotels.
When we apply business intelligence in the context of hotel sales, marketing and revenue management, it makes sense that BI can lend the most support to considerations that depend on data-intensive calculations that are beyond the scope and capability of a hotel’s systems and processes. One such area is understanding booking pace – that ubiquitous determination of what is sold (“on the books”) for a future period, compared to what was sold for the equivalent period at the same time last year. This is considered a critical measure in most hotels and hotel companies, and many go to great lengths to regularly compile this indicator with differing degrees of granularity.
And while business intelligence can greatly assist in this area by automating the continual calculation of hotel booking pace at very granular levels (for instance, down to the level of a single rate program or negotiated account) and in non-traditional ways (viewing booking pace by channel, room type or source market for example), perhaps the biggest advantage that BI brings is in providing the means to help interpret booking pace. Here we will consider three relevant areas in which hotel business intelligence can help to assess what a measure of booking pace really means for a hotel:
Increases in booking pace should be interpreted relative to forecasted demand
At the market segment level, group booking pace is often monitored closely, with increases over the prior year encouraged and rewarded. Though it is true that group booking pace being up is likely an indicator of increased sales manager engagement/performance, whether or not this state is desirable for a given future period depends largely on forecasted demand for that period and group rates relative to the forecasted demand of other segments. Even at the house level – and even with year-over-year ADR growth – increases in booking pace are not always desirable. Selling out a given date range prematurely can lead to missed late-booking opportunities, and this can translate into market share declines. BI can help by providing the means to continually monitor booking pace (and remaining capacity) at the necessary level of detail, for instance by market segment. Manual processes (such as manually updated Excel models) fall short here due to the data-intensive nature of the required calculations.
Declines in booking pace should be interpreted relative to changes in lead time
Apart from the insinuation of the paragraph above, there are other reasons why a decline in booking pace is not necessarily cause for alarm. One such consideration is the lead time of bookings (how far in advance bookings are reserved prior to arrival), specifically reductions in lead time year-over-year. As we know, there are many factors that affect how far in advance guests book, from the general economic climate to specific circumstances in feeder markets (cultural, political or economic), to the impact of technological advances such as mobile booking devices and platforms. When we can measure and understand lead time with great precision (which is accomplished through the application of hotel business intelligence), then we can better interpret booking pace.
A year-over-year reduction in lead time (be it general or for a given market segment or feeder market) would manifest itself as a decline in booking pace. For instance, we wouldn’t have as many rooms on the books for the upcoming month as we did at this time last year. However, if we knew that this was merely the result of shortened lead times (for whatever reason) then we would know to not be alarmed (the business will materialize; it will just book closer to arrival in accordance with the observed trend). Our actions would reflect this calm, and would likely be very different from our actions if we had only noted a decline in booking pace without observing the trend in lead times. Also note that as opposed to the “natural” factors listed above, there may also be “manufactured” factors (such as our own pricing/promotional tactics) that affect lead time. Here too, the application of business intelligence provides the means to assess those factors and make informed decisions.
Booking pace itself has a pace that should be monitored and understood
A bit trickier to grasp (but still very sensible) is the concept of the “pace of booking pace”. What we mean here is that booking pace at any point in time for a given future date range is a dynamic measurement. What we have on the books now for next month relative to the same measure one year ago provides us with only a singular data point. This data point itself is meaningful, but we should also understand how it is changing over time. What was that data point (the booking pace surplus/deficit) four weeks ago for the same upcoming month? What was it two weeks ago? From this series of data points, is the booking pace for the upcoming month increasing or decreasing? If we use business intelligence to help us measure and track booking pace – and the pace of booking pace – then we can create more accurate hotel forecasts and make better revenue management, sales and marketing decisions.
These are some of the primary considerations that should be taken into account when interpreting the booking pace of a hotel or hotel portfolio. No doubt, this list could be expanded to include other areas of consideration. The applicability of business intelligence to the interpretation of hotel booking pace is quite clear: the data-intensive nature of the booking pace calculation itself (when computed continually and with an optimal level of granularity) necessitates such supportive processes and technology. Add to this calculation the proximal factors that increase the accuracy of our interpretation of booking pace – such as forecasted demand, lead time and the pace of booking pace itself – and we likely (hopefully) won’t look at booking pace the same way again.
Comments